PALMs
Perceptual-Adaptive Learning Modules (PALMs) are web-based learning aid software based on Cognitive Science principles. PALMs intend to address crucial aspects of learning—such as pattern recognition—that traditional instruction methods have overlooked.
Figure1: In PALMs, the user classifies novel presentations into appropriate groups, such as diagnoses, according to their underlying features. The adaptive learning algorithms used by PALMs schedule learning items based on the individual’s performance. These algorithms are unique in using both response time and response accuracy as ongoing measures of learning strength.
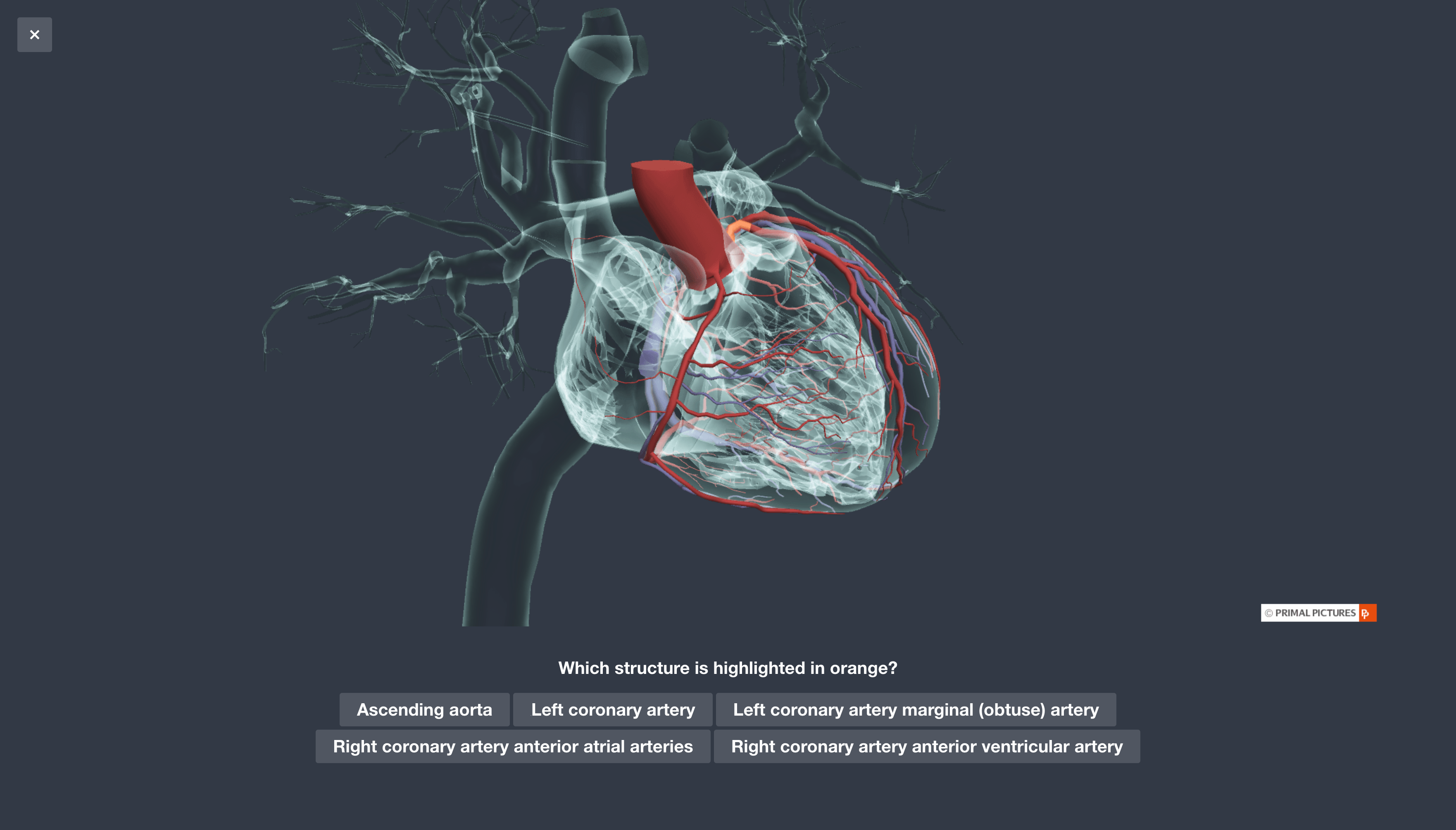
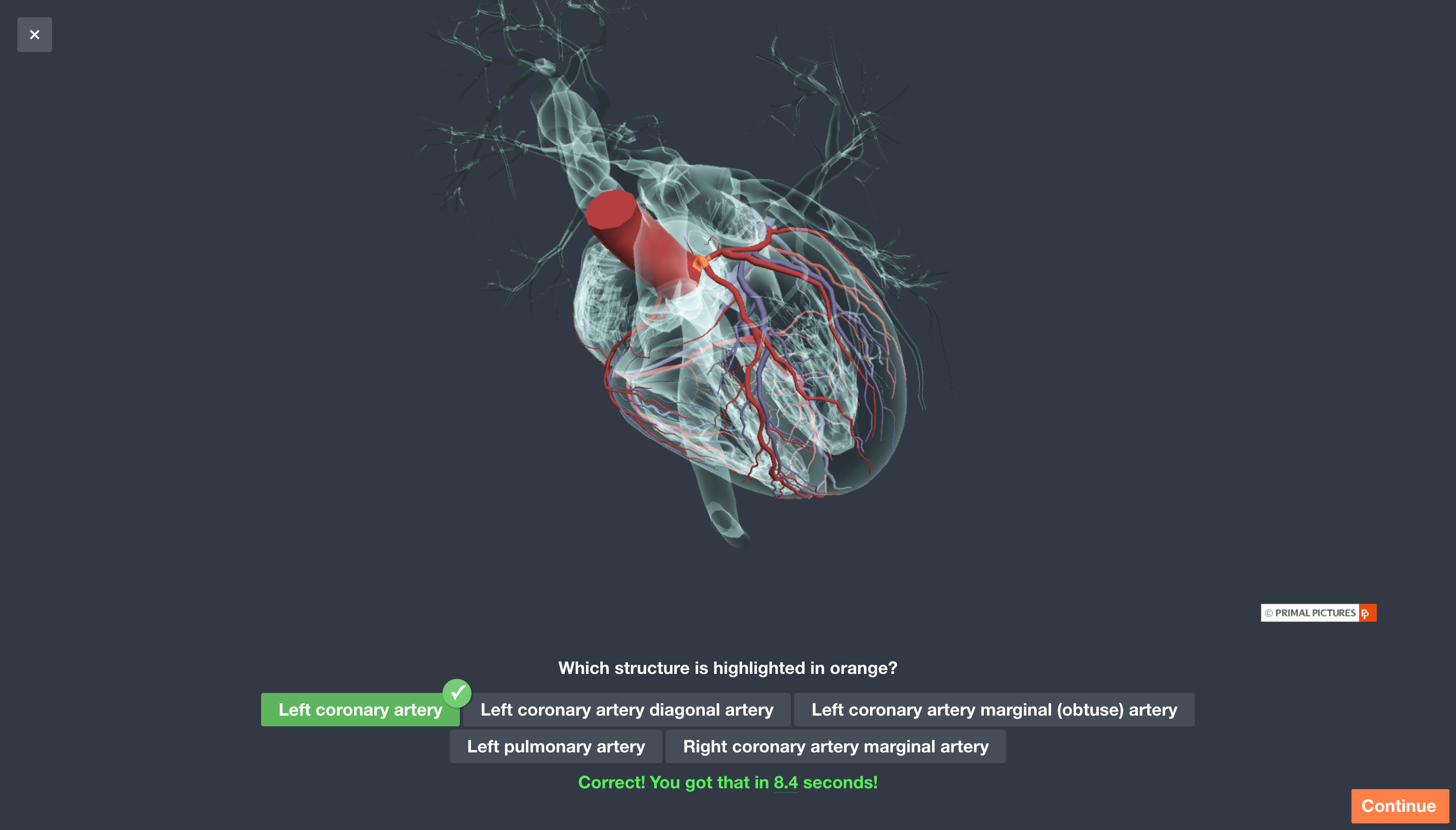
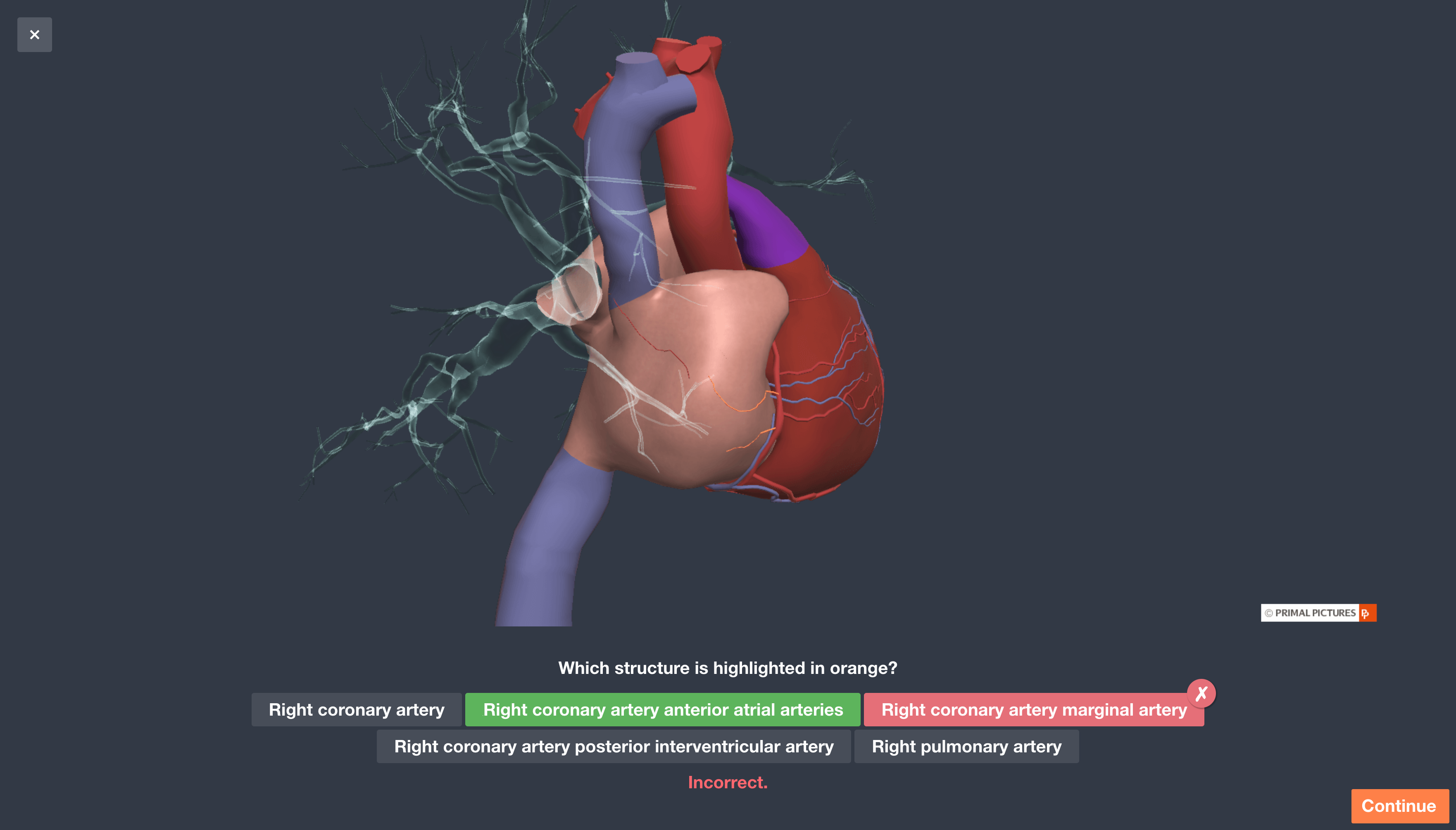
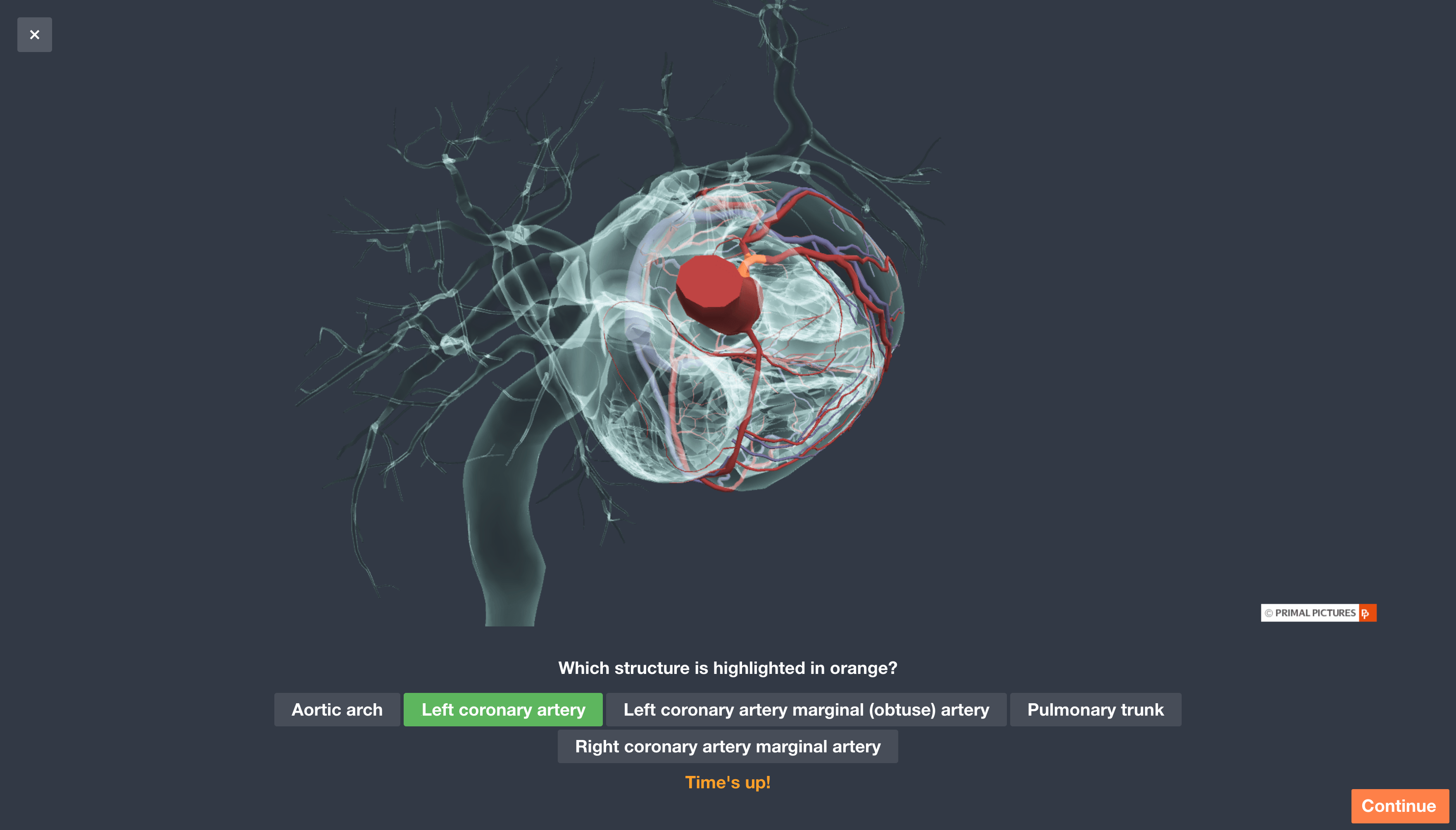
Figure1: In PALMs, the user classifies novel presentations into appropriate groups, such as diagnoses, according to their underlying features. The adaptive learning algorithms used by PALMs schedule learning items based on the individual’s performance. These algorithms are unique in using both response time and response accuracy as ongoing measures of learning strength.
Metadata
Target Audience
Medical students and residents
Medical students and residents
Platform
Responsive Web App
Responsive Web App
Team
Domain Experts x 3
Project Manager x 1
Engineer x 3
Designer x 1 👋
Domain Experts x 3
Project Manager x 1
Engineer x 3
Designer x 1 👋
Timeline
Initial development in 2014 with continuous optimization since and various iterations with different subjects
Initial development in 2014 with continuous optimization since and various iterations with different subjects
Objective
This was initially a pilot product to help the company pivot from K-12 math to medical education. The goal was to build our first medical module.
Problems
The core technology and development framework were there when I joined the team. But, many user-facing aspects of the product had issues (e.g., sub-optimal legibility, poor touch-screen usability, ambiguous progress indicators, lack of onboarding, dated visual language, content-related anomalies, discouraging user experience, etc.). Additionally, since the team had been iterating on the concepts for years, the framework had accumulated poor practices and outdated libraries, making solution implementation complicated.
My Role
As the first and only design hire, my role was to partner with our main domain expert—to materialize her vision—while working closely with the engineers to redesign the interfaces and address usability issues.
Example Contribution
Standardization
To streamline the development process for PALMs and improving the user experience for the learners, I introduced a new components that includes many visual and behind-the-scenes patterns.
Figure 2: A selection of patterns from the new design system such as core problem presentation stracuture, icon design, and messaging inserts.
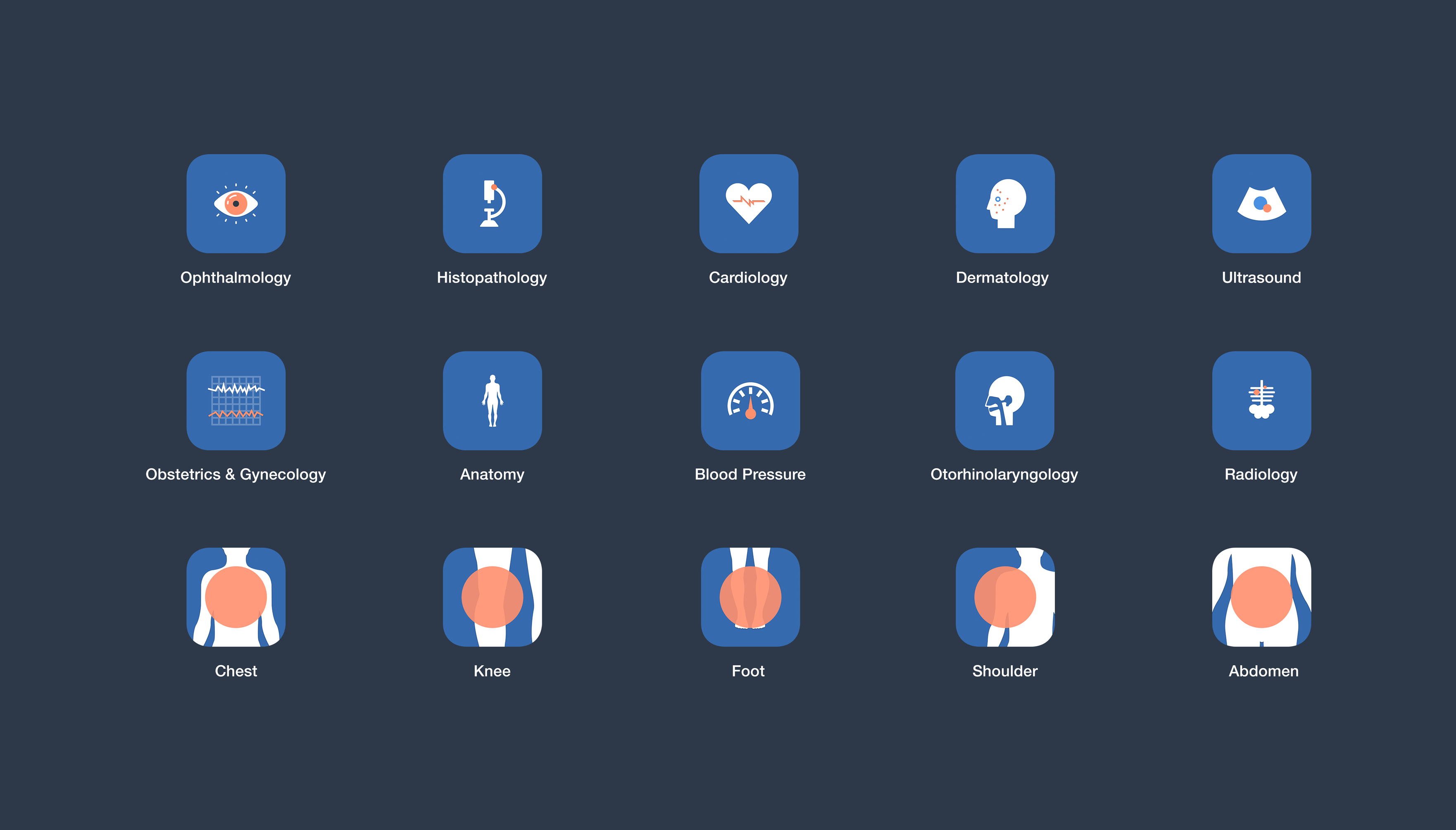
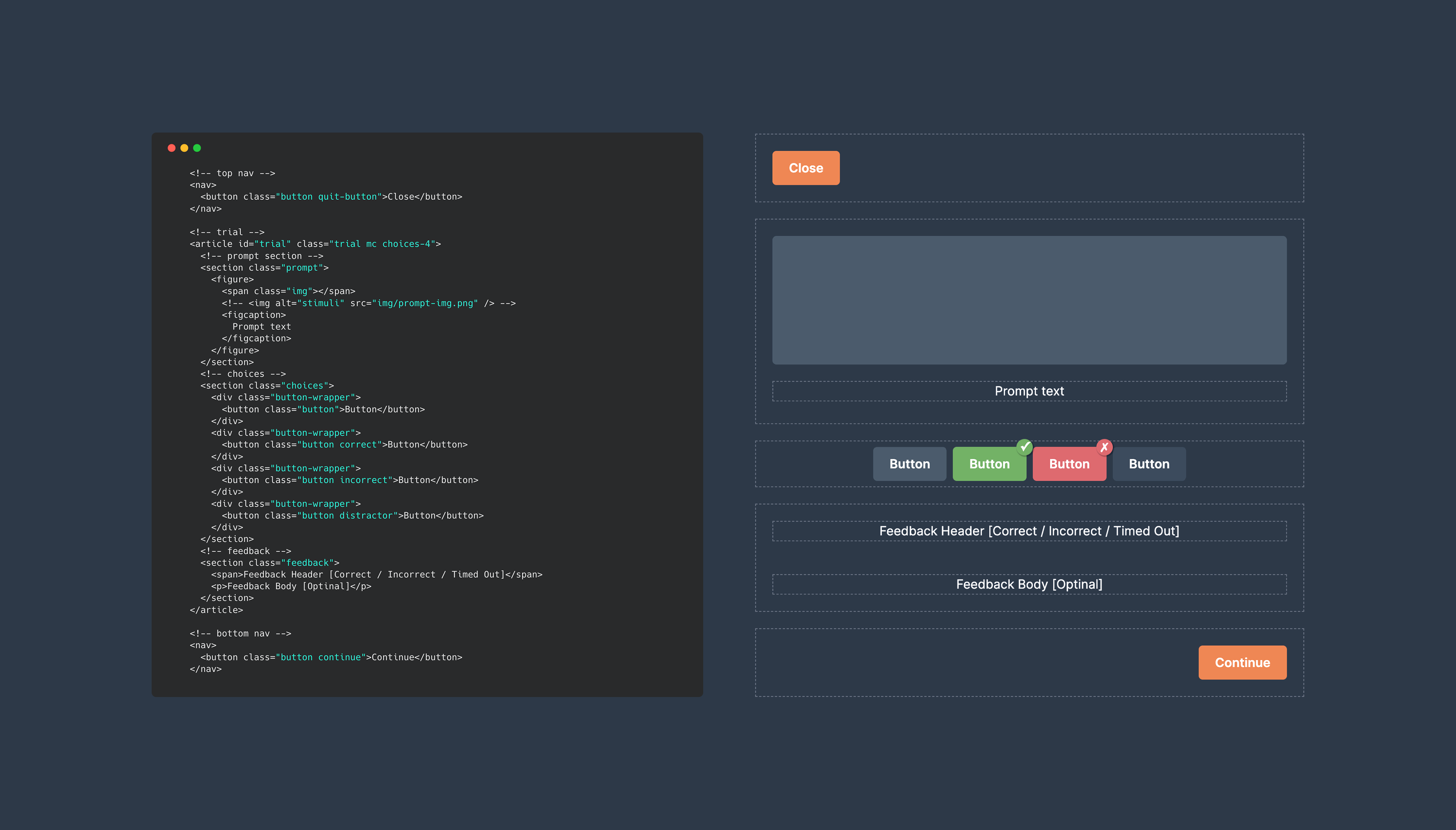

Figure 2: A selection of patterns from the new design system such as core problem presentation stracuture, icon design, and messaging inserts.
Example Contribution
Enhanced Feedback Cues
To ensure the learning feedback cues are universally usable and understandable, and quick to parse
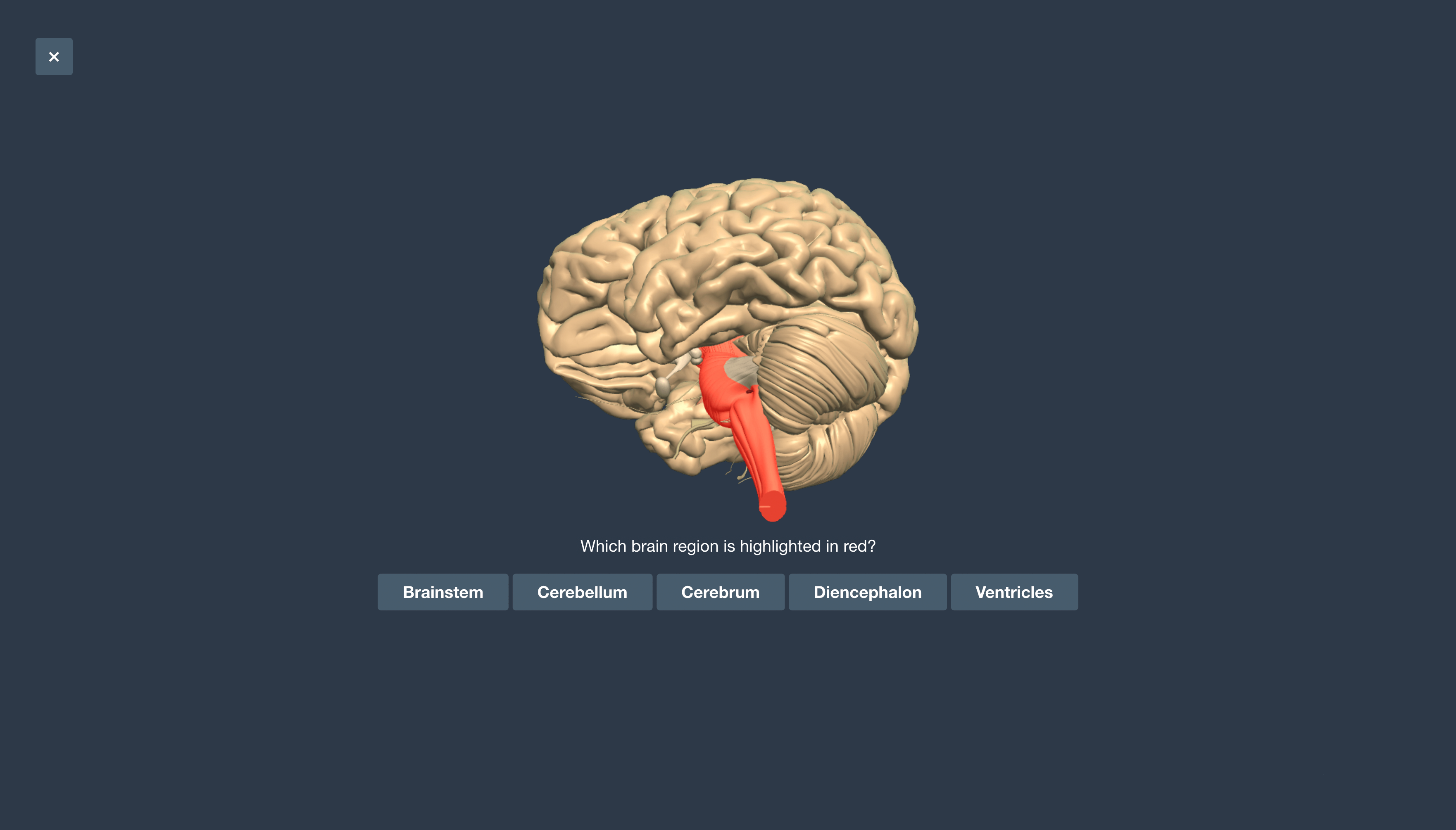
Figure 3: A Brain Regions problem in prompt state; the problems are timed but to resude response presure, no timer is included. This decision is based on previous user suveys.
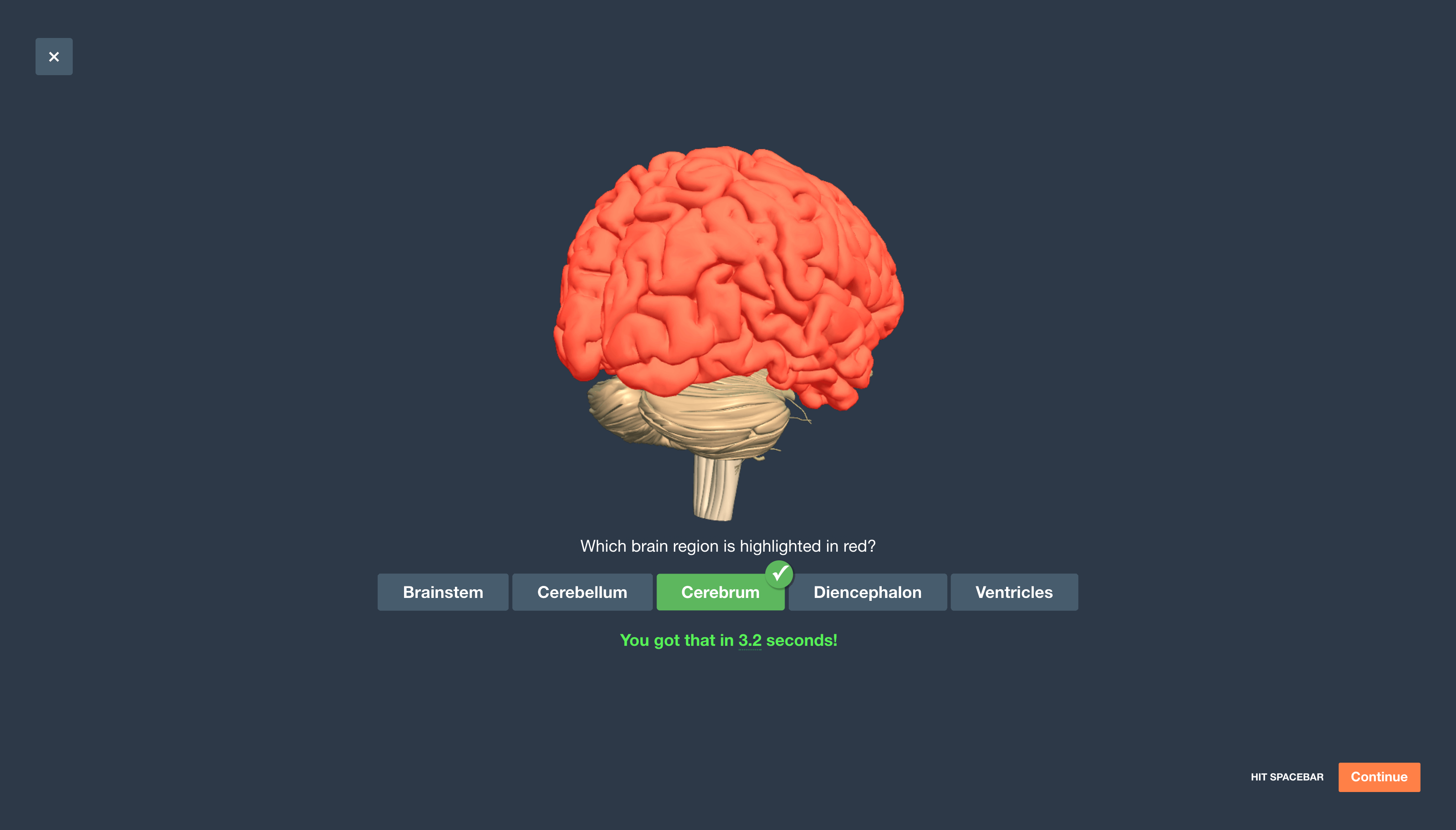
Figure 4: A problem in feedback state, displaying correct response cues, including the response time
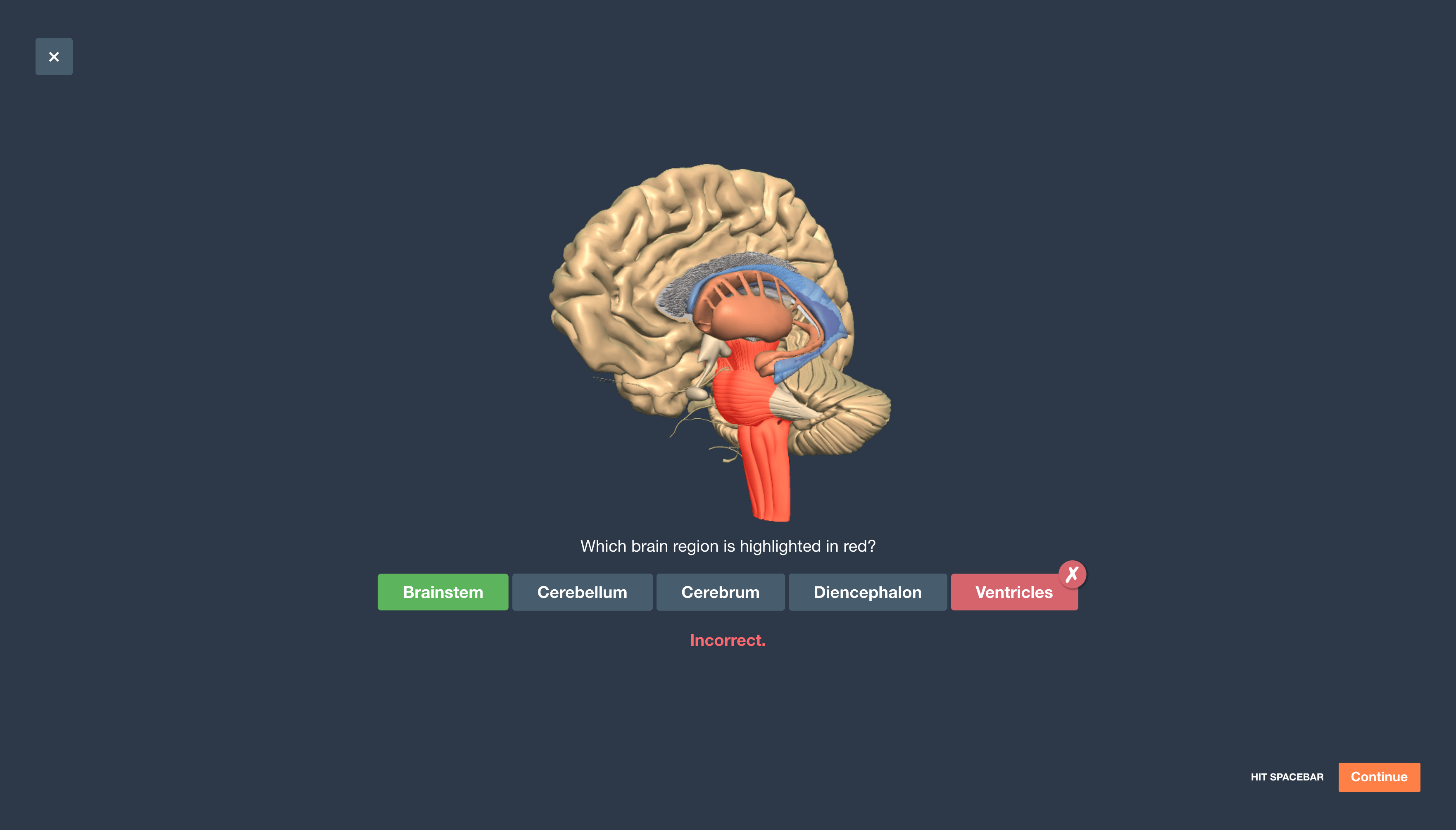
Figure 5: A problem in feedack state, displaing incorrect as well as the correct response cues; since response time for incorrect inputs are irrelevant, the response time is not shown.
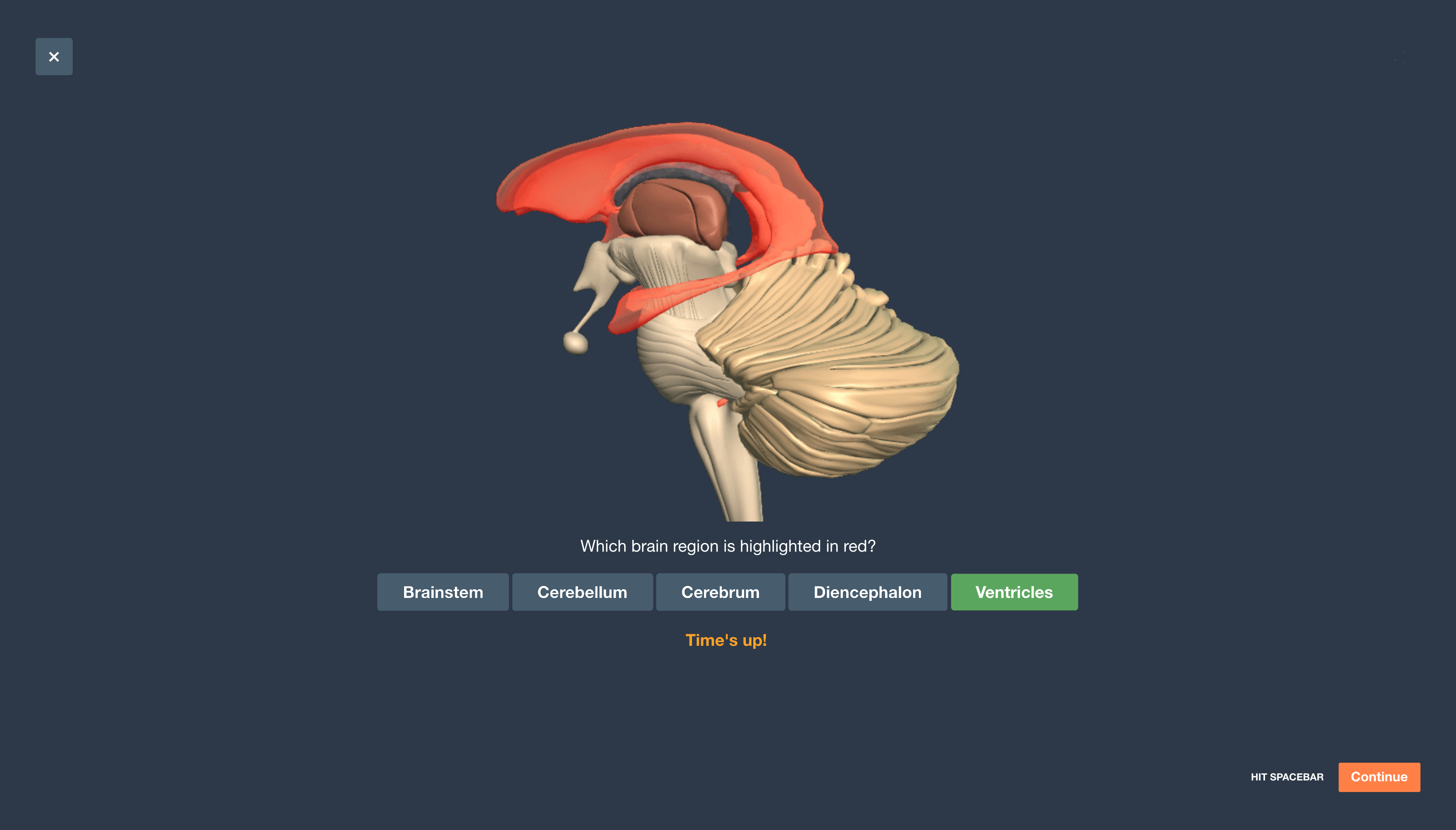
Figure 6: A problem in timed-out state displaying the missed correct response
Example Contribution
Progressive Disclosure
I revised the general flow and inserted new nodes to provide dedicated real estate for timely hand-holding and way-finding indicators. These inserts host static and dynamic messages that aid users build an accurate mental model of the journey.
![]()
Figure 7: The comparison of the original flow (first row) with the evolved one (second row).
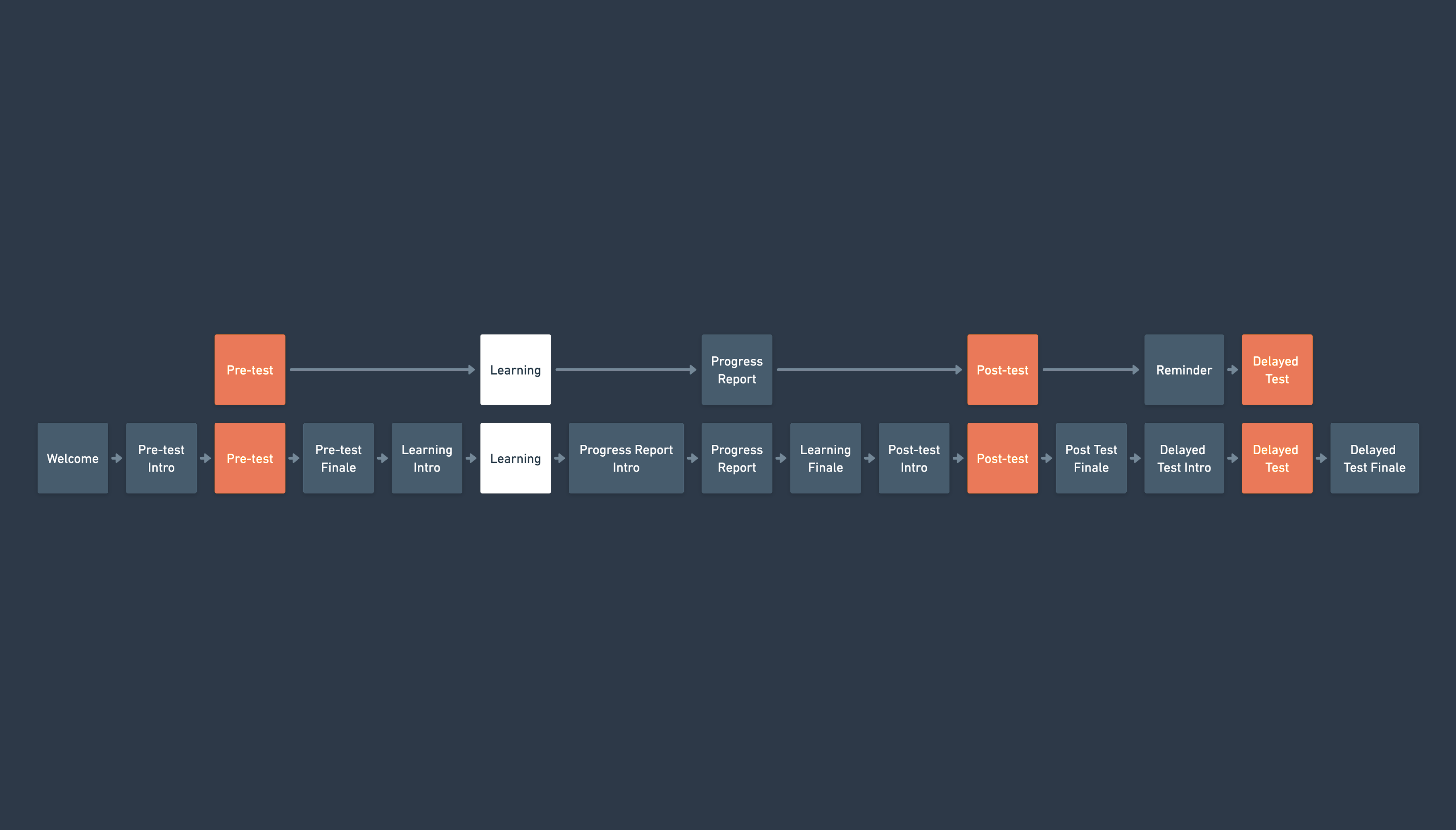
Figure 7: The comparison of the original flow (first row) with the evolved one (second row).
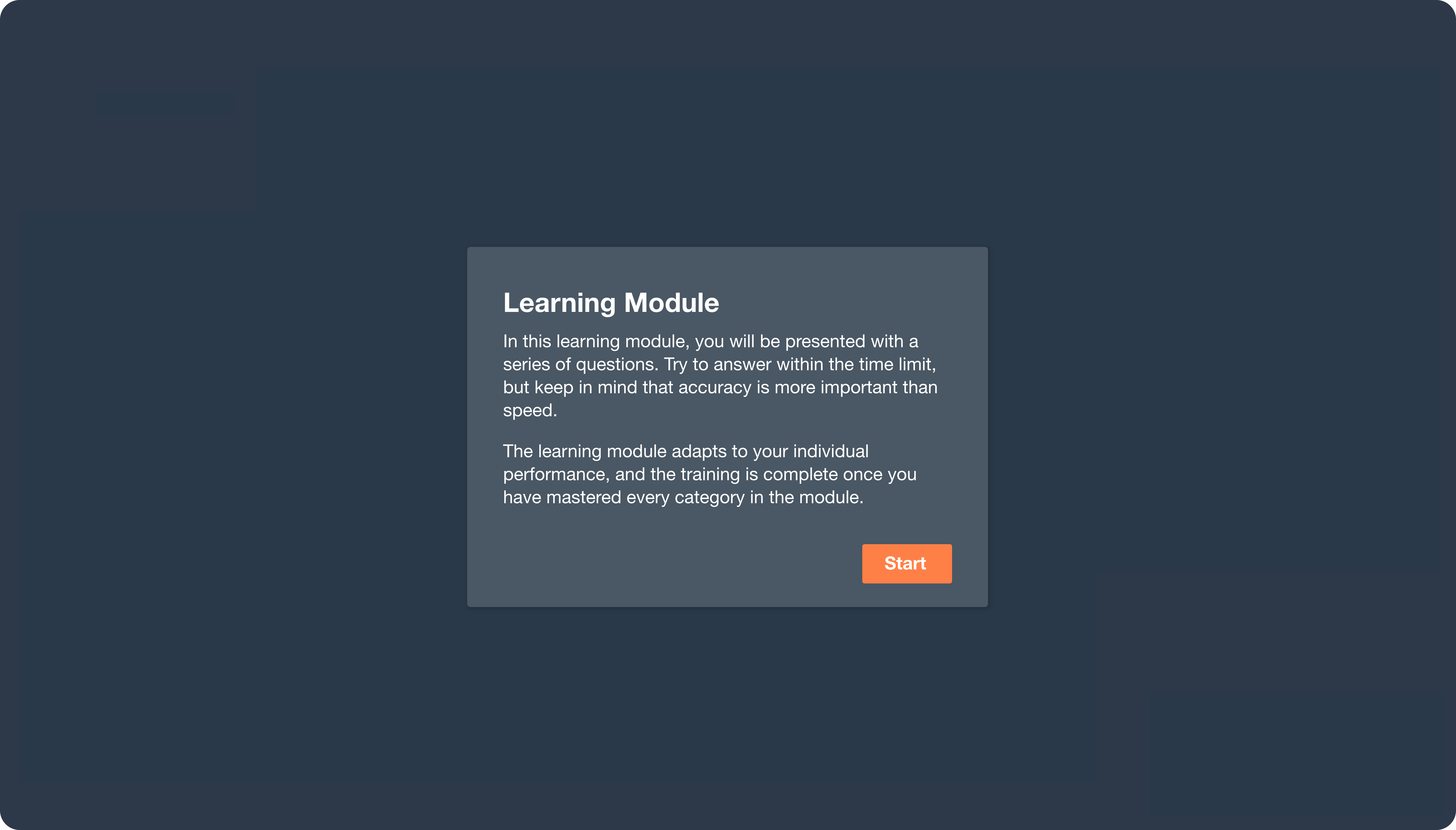
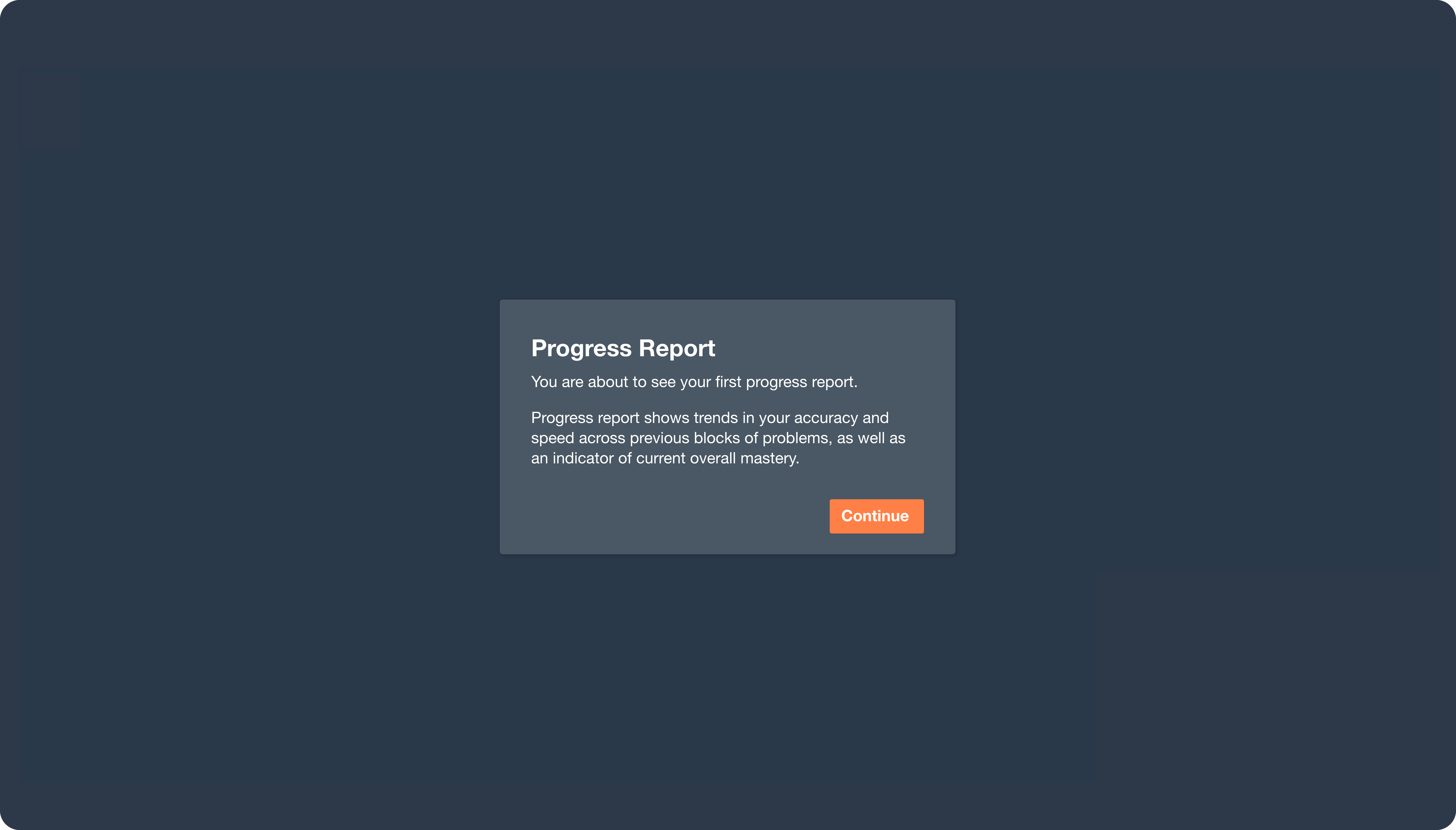
Example Contribution
Messaging & Positioning
To communicate the technical behind-the-scenes aspects of the product, I co-scripted and created an explainer video, helping novice users understand PALMs.
Figure 10: An explainer video on the product's value proposition; I established a new workflow for creating videos that follow these steps: co-scripting, storyboarding and scene creation, defining the visual language, and animation execution.
Figure 10: An explainer video on the product's value proposition; I established a new workflow for creating videos that follow these steps: co-scripting, storyboarding and scene creation, defining the visual language, and animation execution.
Outcome
PALMs have helped many medical students, trainees, and professionals develop deep understanding and automaticity with complex subjects. The efficiency and effectiveness of PALMs in training recognition and interpretation of clinical tests and maintaining this training over many months has been demonstrated in published research[1][2][3][4] and presentations at meetings targeting both clinical specialties and medical education.
- Krasne S, Stevens CD, Kellman PJ, Niemann JT. Mastering Electrocardiogram Interpretation Skills Through a Perceptual and Adaptive Learning Module. AEM Educ Train. 2020;5(2):e10454. Published 2020 May 5. doi:10.1002/aet2.10454
- Romito BT, Krasne S, Kellman PJ, Dhillon A. The impact of a perceptual and adaptive learning module on transoesophageal echocardiography interpretation by anaesthesiology residents. Br J Anaesth. 2016;117(4):477-481. doi:10.1093/bja/aew295
- Rimoin L, Altieri L, Craft N, Krasne S, Kellman PJ. Training pattern recognition of skin lesion morphology, configuration and distribution. Journal of the American Academy of Dermatology.
- Krasne S, Hillman JD, Kellman PJ, Drake TA. Applying perceptual and adaptive learning techniques for teaching introductory histopathology. J Pathol Inform. 2013;4:34. Published 2013 Dec 31. doi:10.4103/2153-3539.123991